Last week, Fero CEO Berk Birand spoke at the Association for Iron and Steel Technology's Digital Transformation Forum on how explainable machine learning can help steel manufacturers solve core business and technical challenges. Here are some takeaways from the conversation:
With the ability to learn and improve in real time, machine learning solutions outperform Six Sigma and SPC.
Most steel plants face the same business challenge: reducing raw material costs to stay competitive, without jeopardizing product quality. Traditional process improvement tools aim to help them achieve this goal, yet they have several key limitations.
Specifically, these tools tend to plan conservatively for the worst-case scenario, i.e. a particular composition of scrap. However, Birand noted that the frequency of variation in steel production—especially at the scrap level—means that this scenario rarely happens. As a result, the tools "don't perform as well as they could."
A machine learning solution, however, adapts continuously to changes on the factory floor. It's like designing an experiment that covers your entire production line multiple times a day—a task that would be impossible with Six Sigma.
Moreover, as Birand put it, "Traditional tools don't do a great job of combining data from across the plant in an end-to-end process." In contrast, machine learning can rapidly process complex correlations and help you make smarter business decisions—as long as you choose an explainable solution.
Machine learning delivers measurable ROI, from lower raw material costs to improved quality.
"With any kind of digitalization project," Birand asked, "the first question should be: How big is the opportunity?"
To demonstrate the size of the opportunity here, he shared some key results from steel plants deploying Fero's explainable machine learning software. Within six months of deployment, the average plant saw alloy reduction corresponding to $1-$6 per ton in raw material savings.
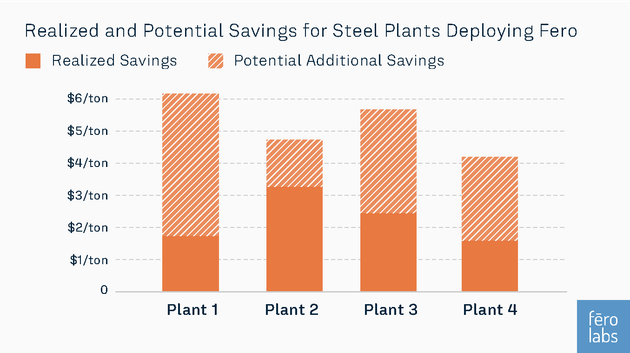
Scaled across five or more plants, the results were even more dramatic, highlighting the vast amounts steel manufacturers could potentially save by deploying Fero across a wider number of grades.
"What our customers are able to do is look at individual plants and compute the potential savings that are possible in terms of raw material reduction," Birand explained. "Over the next year, if you deploy machine learning over every heat and every product grade, this is how much you'll save."
Machine learning lets you optimize your operations in real time.
While machine learning has many advantages over Six Sigma, it doesn't contradict its core principles. In the context of the traditional DMAIC cycle, you can think of machine learning as a vastly more powerful "Analyze," continuously learning and improving in the background.
Predictions and root cause analysis are two key benefits of this real-time analysis, and it doesn't end there. A machine learning solution can even tell you what raw material additions and process parameter settings will minimize costs, while ensuring that you still meet quality targets. This has implications on the entire workflow, as quality and operations groups can confidently change production settings while the product is still being processed—improving the bottom line.
Birand pointed to alloy reduction as one case where steel plants have particular need for real-time machine learning.
"We can open up the alloy bounds to have a machine learning system adapt the alloy specs in real time," he explained. "The system can reduce alloy consumption for the average production situation, leading to a decrease in raw material costs. But when things are actually worst-case, these systems are smart enough not to make any changes."