Industrial engineers have long relied on traditional methods for conducting root cause analyses (RCA) without the use of AI. These time-tested approaches have proven effective in identifying and addressing issues in manufacturing processes, but they also come with certain limitations.
Let's explore the conventional RCA process used by manufacturers and compare it to AI-powered solutions like Fero Lab's Root Cause Explorer.
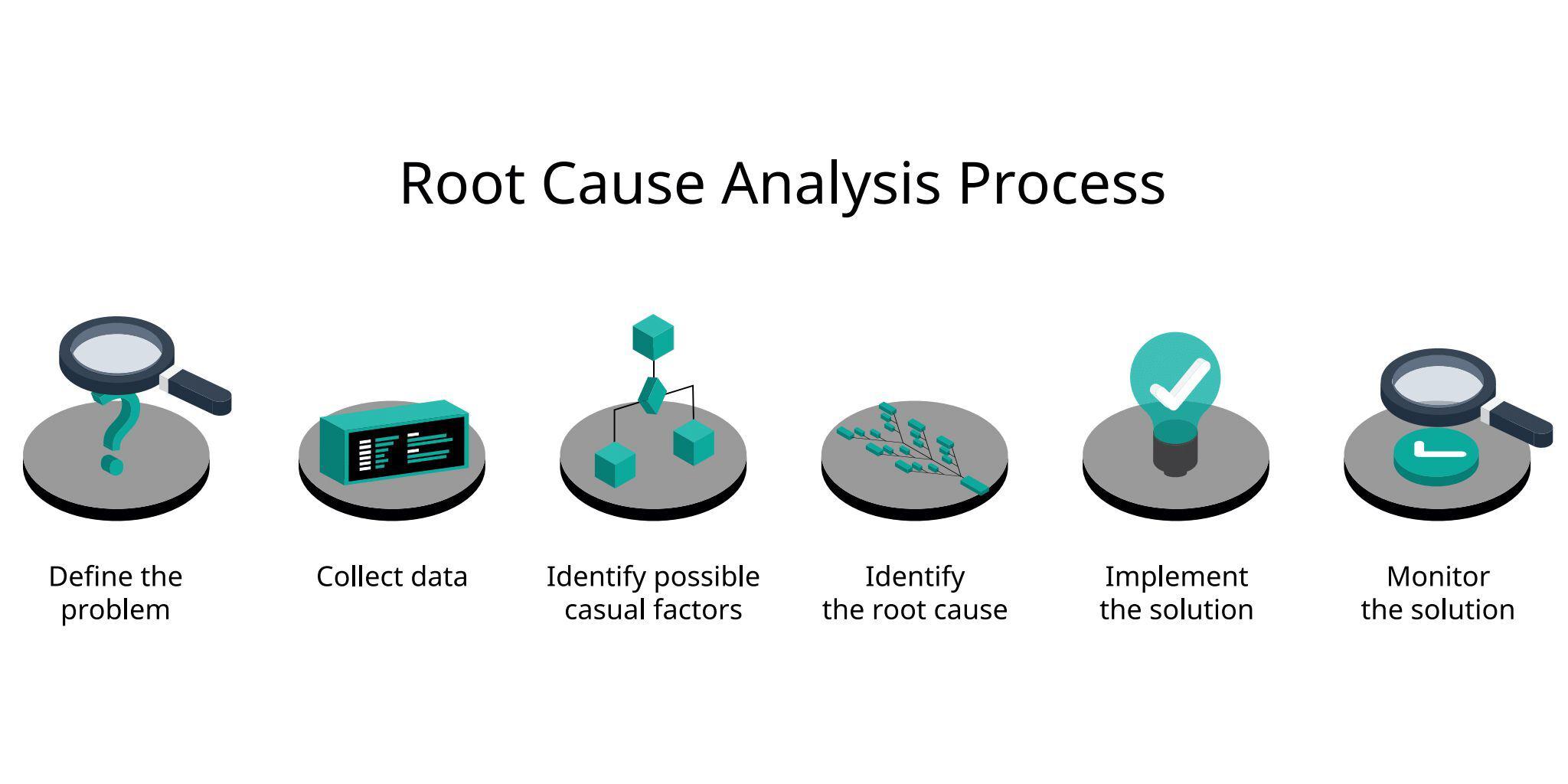
Traditional Root Cause Analysis Process
Industrial engineers typically follow a structured approach to root cause analysis that involves several key steps:
1. Problem Identification: Engineers clearly define the problem or defect they want to solve, using data and facts to support their statement.
2. Data Collection: Relevant data is gathered from various sources, including sensors, manufacturing software, production logs, and quality control reports.
3. Cause Identification: Engineers use tools like the fishbone diagram (Ishikawa diagram) to visually organize and categorize potential causes of the problem.
4. Analysis: Techniques such as the 5-Why method are employed to dig deeper into root causes, not just symptoms or immediate causes.
5. Solution Generation: The team brainstorms and evaluates different options to address the identified root causes.
6. Implementation and Evaluation: Chosen solutions are implemented, and their effectiveness is measured and verified using data and feedback.
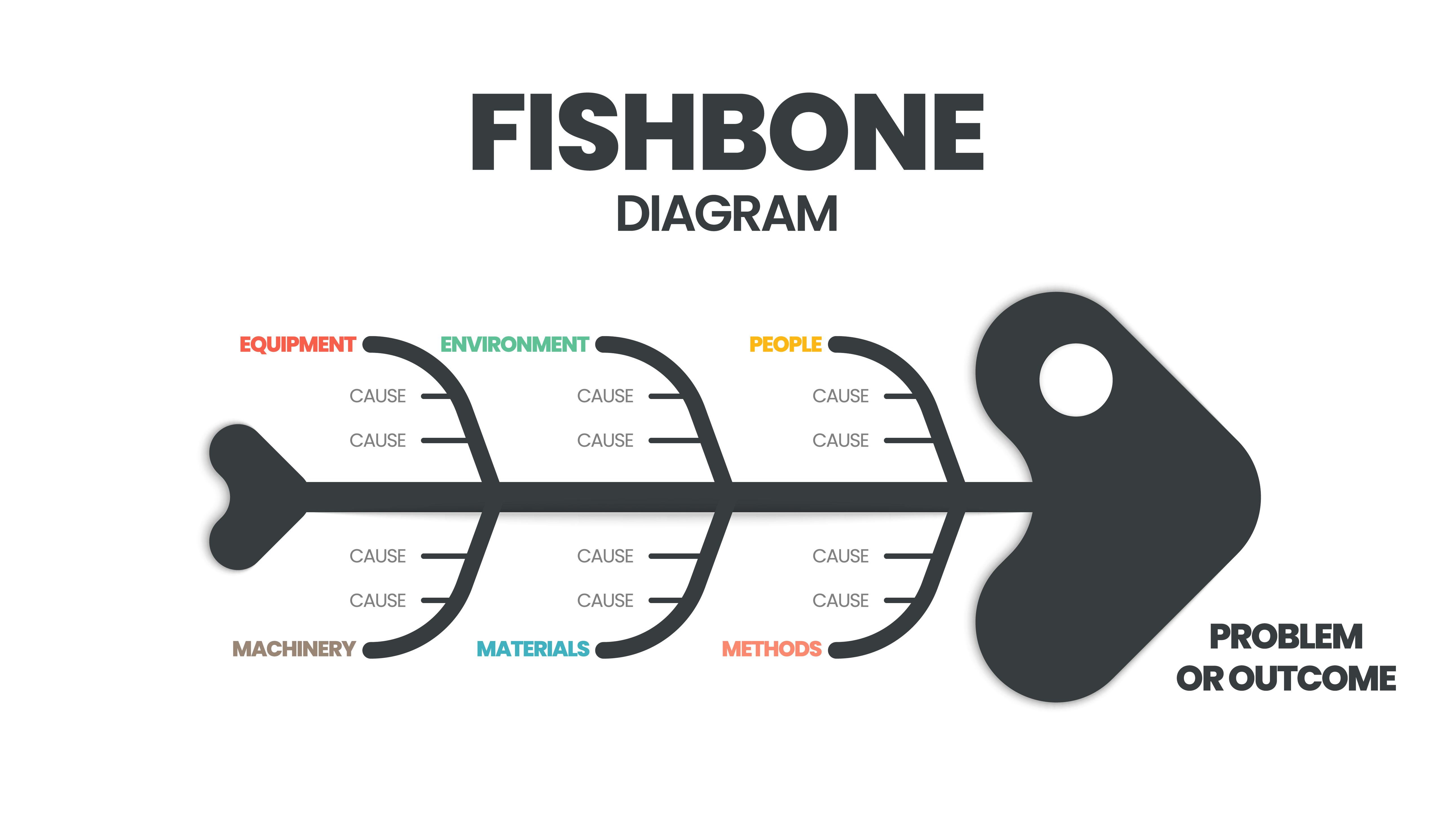
Strengths of Traditional RCA
- Human Expertise: Leverages the knowledge and experience of skilled engineers and subject matter experts.
- Flexibility: Can be adapted to various types of problems and industries.
- Team Collaboration: Encourages cross-functional teamwork and diverse perspectives.
- Established Methods: Uses well-known tools like 5-Why, fishbone diagrams, and FMEA that have proven effective over time.
Weaknesses of Traditional RCA
- Time-Consuming: Manual data collection and analysis can be lengthy, potentially leading to extended downtime.
- Human Error: Prone to biases, oversights, and mistakes, especially under time pressure.
- Limited Data Processing: Humans struggle to process and analyze large volumes of complex data quickly.
- Inconsistency: Results may vary depending on the team's composition and individual expertise.
- Resource Intensive: Requires a team of experts with diverse skills, which can be costly to maintain.
AI-Powered Root Cause Analysis
AI solutions like Fero Labs offer a new approach to root cause analysis in industrial engineering. These systems use advanced algorithms and machine learning to analyze vast amounts of data and identify causal relationships.
Advantages of AI-Powered RCA
- Speed: AI can process enormous volumes of data in real-time, significantly reducing the time required for analysis.
- Accuracy: By relying on objective data rather than human interpretation, AI reduces the risk of bias and human error.
- Complex Pattern Recognition: AI excels at identifying intricate relationships between various manufacturing parameters that humans might miss.
- Continuous Learning: AI systems can continuously update their models as new data becomes available, improving accuracy over time.
- Predictive Capabilities: Fero Labs Root Cause Explorer can not only identify root causes but it will also predict potential issues before they occur.
- Resource Efficiency: AI-powered RCA reduces the need for large teams of experts to get involved, potentially lowering costs and keeping workers focused on their usual tasks.
- Prescriptive Recommendations: In addition to finding root causes and predicting potential issues before they occur, Fero Labs' Root Cause Explorer will recommend what to do to resolve the issue.
Traditional root cause analysis methods used by industrial engineers have proven effective but can be time-consuming and resource-intensive. Industrial AI-powered solutions like Fero Labs offer significant advantages in terms of speed, accuracy, and the ability to handle complex data relationships. However, the ideal approach likely combines the strengths of both methods – leveraging AI's data processing capabilities while still incorporating human expertise and contextual understanding.
As manufacturing processes become increasingly complex and data-driven, the integration of AI in root cause analysis is becoming not just beneficial but necessary for maintaining competitiveness and efficiency in industrial engineering.
While challenges exist in implementing these new technologies, the potential benefits in terms of reduced downtime, improved quality, and enhanced decision-making make AI-powered RCA an increasingly attractive option for forward-thinking industrial engineers.